Submit a Manuscript to the Journal
Applied Artificial Intelligence
For an Article Collection on
Primary Theoretical and Practical Applications and Limitations of Generative Artificial Intelligence
Abstract deadline
27 October 2024
Manuscript deadline
19 January 2025
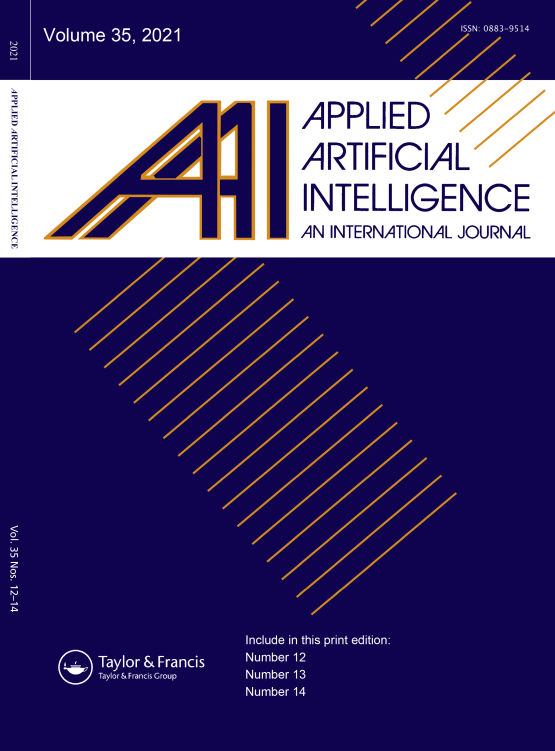
Article collection guest advisor(s)
Dr. Silvia García Méndez,
University of Vigo
[email protected]
Dr. Francisco de Arriba-Pérez,
University of Vigo
[email protected]
Primary Theoretical and Practical Applications and Limitations of Generative Artificial Intelligence
In recent years, Generative Artificial Intelligence models have emerged with promising capabilities. For instance, models like Google Bard, ChatGPT, DALL-E, and Midjourney allow the creation of virtual assistants, summarizing texts, detecting entities, and automatically creating images.
However, generative artificial intelligence is not free from limitations and challenges that have yet to be explored. These models lack memory, are too heavy for use on conventional computers and servers, and exhibit privacy risks. Furthermore, their predictions need to be traced, which limits their ability to explain and interpret and reduces users' confidence in them. Moreover, the data sets used for training may also be subject to bias while at the same time lacking enough global context knowledge, that is, information for particularisation and personalisation.
Although the potential of generative artificial intelligence models is evident, their limitations still need to be addressed. Finding solutions that include short- and long-term memory allows us to personalize and contextualize the system responses. Reducing the hidden layers would allow us to make on-demand models executable on machines with limited resources and increase privacy by limiting access by third-party entities. Furthermore, achieving explainable models would allow end users to understand the nature of the decisions made by these systems. Image-generating systems are a representative example. Tagging the sources contributing to a new picture can significantly streamline assigning specific copyrights. This paves the way for effective regulation and monetization of human content creators, who may perceive these systems as a threat rather than an opportunity.
This call aims to collect original articles focusing on the primary limitations of generative artificial intelligence models, not only practical (e.g., context and memory management, layer pruning, etc.) but also theoretical (e.g., data privacy through encrypting methodologies), towards ethical and cognitive, self-awareness AI.
These works must analyse the limitations and feasible solutions. Thus, both practical and theoretical works that explore the hidden layers, provide alternatives for traceability, and develop applications in relevant and multidisciplinary use cases will be considered.
Topics considered for this Call for Papers include, but not limited to, the following:
• Lack of memory in Large Language Models (LLM)
• On-demand generative artificial intelligence models
• Pruned models
• LLM encryption
• Privacy in generative artificial intelligence
• Explain and interpret in generative artificial intelligence
• Personalized generative artificial intelligence models
• Engineering applications of LLMs
• Generative artificial intelligence in multidisciplinary use cases
This Article Collection also welcomes contributions on new trends in Generative Artificial Intelligence
Keywords: Generative Artificial Intelligence, Large Language Models, Natural Language Processing, Human Computer Interaction, GAI Case Studies
Please review the Submission Instructions below prior to submitting an abstract and/or manuscript.
Article Collection Guest Advisors
Dr. Silvia García-Méndez received her Ph.D. in Information and Communication Technologies from the University of Vigo, Spain, in 2021. Since 2015, she has worked as a researcher with the Information Technologies Group at the University of Vigo. She is collaborating with foreign research centers as part of her postdoctoral stage. Her research interests include Natural Language Processing techniques and Machine Learning algorithms.
Dr. Francisco de Arriba-Pérez received his Ph.D. in 2019 from the University of Vigo, Spain. He is currently a researcher in the Information Technologies Group at the University of Vigo. His research includes the development of Machine Learning and Natural Language Processing solutions for different domains like finance and health.
Benefits of publishing open access within Taylor & Francis
Global marketing and publicity, ensuring your research reaches the people you want it to.
Article Collections bring together the latest research on hot topics from influential researchers across the globe.
Rigorous peer review for every open access article.
Rapid online publication allowing you to share your work quickly.
Looking to Publish your Research?
Find out how to publish your research open access with Taylor & Francis Group.
Choose open accessSubmission Instructions
Abstract Submissions:
Abstract submission is open until 27th October 2024.
Please carefully review the journal scope and author submission instructions prior to submitting an abstract as it will be rejected if it does not fall within the scope of the journal.
Please send your abstracts by email to Christopher Montgomery, Commissioning Editor, for further consideration.
Manuscript Submissions:
Manuscript submission is open until 19th January 2025.
All manuscripts submitted to this Article Collection will undergo desk assessment and peer-review as part of our standard editorial process. Manuscripts which do not fall within the scope of the journal will be rejected.
Please be sure to select the appropriate Article Collection from the drop-down menu in the submission system, and to include the 10% Discount code: UAAI-2025-C29055 before you submit your manuscript as discount codes cannot be applied retroactively.
We do have a limited amount of 20% Discount codes only available for those who are able to submit an Abstract. It should be noted that discount codes cannot be combined and that only the higher discount would be applicable.
Please contact Christopher Montgomery, Commissioning Editor, with any other queries and discount codes regarding this Article Collection.
Article Publishing Charges:
The standard article publishing charge (APC) for this journal is $2195/£1756/EUR2110/AUD3060, plus VAT or other local taxes where applicable in your country. There is no submission charge.
Please visit the APC Cost Finder page to find the APC applicable to your specific country and article type.
If you are based at an institution or associated with a funder that has an open access publishing agreement with Taylor & Francis, you might be eligible for APC support. Please see details here.
We offer discounts and waivers for authors in developing countries as defined by the World Bank, either 50% or 100% depending on where the institution of the corresponding author is located. Please see details here.
We will consider requests for discretionary waivers from researchers who aren’t eligible under the above policies. Please note that discounts must be applied at the Charges stage of the submission process when the APC quote is confirmed and may not be considered after submission.
If you have any questions about eligibility, please use the Contact Us form.
All manuscripts submitted to this Article Collection will undergo desk assessment and peer-review as part of our standard editorial process. Guest Advisors for this collection will not be involved in peer-reviewing manuscripts unless they are an existing member of the Editorial Board. Please review the journal Aims and Scope and author submission instructions prior to submitting a manuscript.